The combination of quantum computing and artificial intelligence (AI) is expected to dramatically enhance many fields of human activity which we have yet to fully imagine. With adoption of AI technologies across other industries, the need for fast and efficient model training processes only seems to grow. While conventional system architectures work adequately in many scenarios, they severely fall short to complex AI algorithms.
Where quantum computing shines as a futuristic technology is the promise of forever changing speed and efficiency in computations. We can potentially open new doors for harnessing AI through the inherent characteristics of quantum mechanics. In this article, we will look at how quantum computing can improve AI model training with numerous applications, problems, and the possibilities from this fascinating merger.
The Current Landscape of AI Model Training
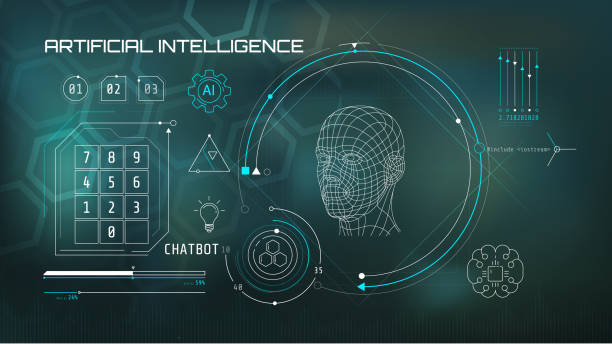
Developing AI models, particularly deep learning ones, is computationally intensive. In the past, enormous datasets were processed using classical supercomputing systems, which is now considered outdated. These systems have downsides like high energy and training duration, and scaling to bigger datasets is challenging. A lot of corporations have poured money into hardware, yet at the pace AI is advancing, their capabilities will soon be insufficient. The state of affairs within the industry calls for a change of approach – quantum computing is the solution. Quantum systems promise not just faster processing times, but also the use of quantum phenomena to solve more complex problems.
How Quantum Computing Works
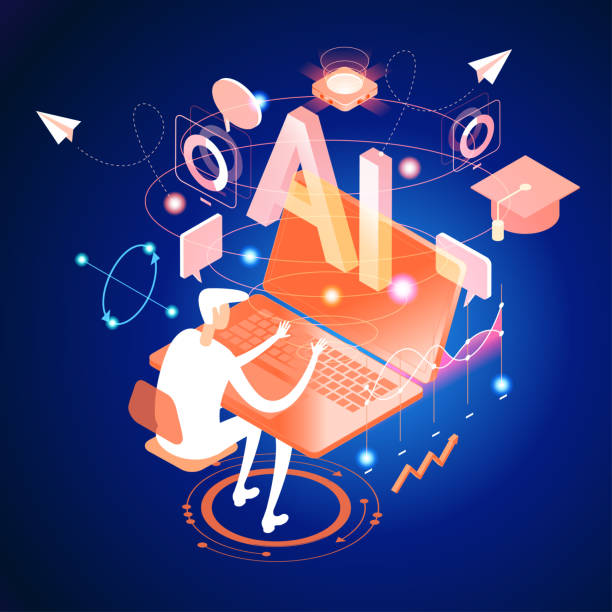
Central to quantum computing are qubits, which differ from classical bits in nature. A classical bit is either a 0 or a 1 but, owing to superposition, a qubit can be many things at once. Moreover, qubits can be entangled, meaning they can be linked even with a great distance between them. This behavior singularly enhances the computational power tremendously for highly complex problems, such as those posed during AI training. Therefore, unlike classical computers that approach problems sequentially, quantum computers tackle multiple problems at once. To appreciate how quantum computing can be integrated within AI systems, grasping these fundamental concepts is key.
Aspect | Classical Computing | Quantum Computing |
---|---|---|
Data Processing | Sequential | Simultaneous |
Bits | 0 or 1 | 0, 1, or both |
Speed | Slower for large datasets | Exponentially faster for complex problems |
Applications | Standard calculations | Large-scale simulations, optimizations |
The Role of Quantum Computing in AI Model Training
The incorporation of quantum computing into the training of AI systems can be beneficial to the process in many ways. In the case of AI, where the scale of the data set is significantly huge, quantum algorithms can be quite useful. Take for example quantum neural networks, they can simultaneously check a large number of possible solutions making their learning process faster and efficient. This level of efficiency would enable classical systems to develop even deeper and complex models which were unfathomable before. With these new levels of computation speed, comes the ability for researchers and developers to build ever changing and ever improving models. This will certainly result in the integration of quantum computing and AI changing the horizon of intelligent systems as we know it.
AI training is one of the most striking utilization when it comes to the utilization of quantum computing and some of the most bracing examples are listed below.
- IBM’s Quantum Experience: A platform that enables users to run quantum algorithms for machine learning applications.
- Google’s Quantum AI Lab: Explores various applications of quantum computing and its potential to enhance AI model training and optimization.
- D-Wave Systems: Focuses on quantum annealing methods that have successfully solved complex optimization challenges relevant to AI.
Challenges and Limitations
The incorporation of quantum computing into AI comes with its set of challenges and one such challenge is the limited availability of quantum hardware, which is still in development. Further, the advanced programming languages needed to operate quantum computers require skills that the bulk of current AI professionals do not have. In addition, there is a need to create more systems capable of combining quantum and classical computing to cater to today’s tech world. Lastly, there is the question of data security and ethics when applying AI powered by quantum systems. Solving these issues will require multidisciplinary collaboration from researchers, engineers, and businesses.
Future Perspectives: The Path Ahead
Integrating AI with quantum computing results in a truly transformative domain awaiting a new paradigm shift. With constant developments, there are expectations of new quantum algorithms designed specifically for AI, which would most likely enhance efficiency and outcomes. Moreover, quantum and classical computing homogenization may lead to hybrid systems that capitalize on the merits of both systems. The growth of programs aimed at training specialists in requisite quantum technology is undoubtedly going to support innovation down the line. Hence, opened up industries, like healthcare, finance, and logistics, would be AI-enable and optimized to new levels and transformed beyond expectation. After all, in pursuing quantum AI, the territory itself wonders with what new inventions and innovations are we ready to achieve further along in the development.
Conclusion
To summarize, the crossover of AI and quantum computing could transform model training in ways that seemed impossible before. The current constraints of traditional models allow for the astounding capabilities of quantum systems to emerge, so furthering the research and development of AI. There are problems that still need to be solved, but the potential in the future is great for those willing to branch out and tackle these new technologies’ challenges. With a new computing era set to emerge, the venture into quantum AI computing is bound to change the very roots of innovation in numerous fields.
Frequently Asked Questions
- What is quantum computing? Quantum computing is a type of computation that utilizes quantum mechanics principles, allowing computers to process information in ways that classical computers cannot.
- How does quantum computing accelerate AI model training? Quantum computing can perform complex calculations faster than classical computers, reducing the time needed for AI model training and enabling exploration of more sophisticated models.
- What are the challenges of using quantum computing for AI? Key challenges include limited access to quantum hardware, the need for specialized programming skills, and the current stage of quantum technology development.
- Are there any successful applications of quantum AI? Yes, some recent research projects have shown promising results in areas such as optimization problems, drug discovery, and complex simulations relevant to AI training.
- What is the future of quantum computing in AI? The future is likely to see more integrated solutions, increased accessibility to quantum technologies, and breakthroughs that could drastically change how AI is developed and utilized.