The development of multimodal AI represents a major leap in the field of artificial intelligence. As the leader of this shift in technology, GPT-4 has the capacity to understand and interpret data ranging from text to images and videos. This ability improves the machine’s comprehension of the world as well as user experiences, making interactions with AI much more natural and interesting. GPT-4 captures a level of AI contextualization unlike any other by integrating multimodal capabilities. This article examines the details of GPT-4 functionalities, emphasizing how the image and video processing capabilities differ from previous versions. We will discuss the numerous sectors that could benefit, the obstacles that must be overcome, and the implications for the future of this revolutionary technology.
What is GPT-4?
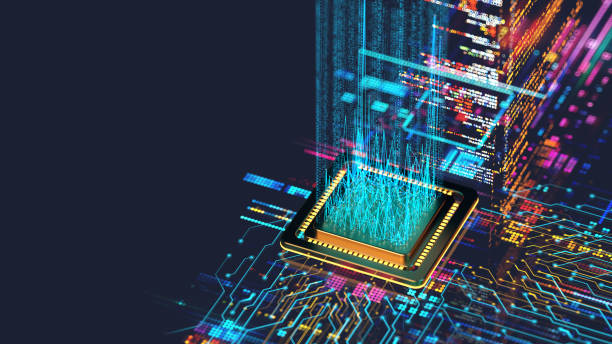
OpenAI has developed an advanced language model known as GPT-4, which is a considerable improvement compared to its predecessors. GPT-4 has additional capabilities compared to earlier versions which focused primarily on text as it can process and understand images. The estimated use cases of the model increases significantly because of this multimodal ability, giving users the ability to interact richer and understand data better. What makes GPT-4 stand out are the contextual awareness features, handling of complex queries, and enhanced fusion of information features. Besides, it has incorporated other methods like reinforcement learning from feedback given by people which improves the quality of the outputs. While reflecting on its features, we have to highlight with a deep concern how this will transform innovation across many industries.
The Significance of Image Processing in GPT-4
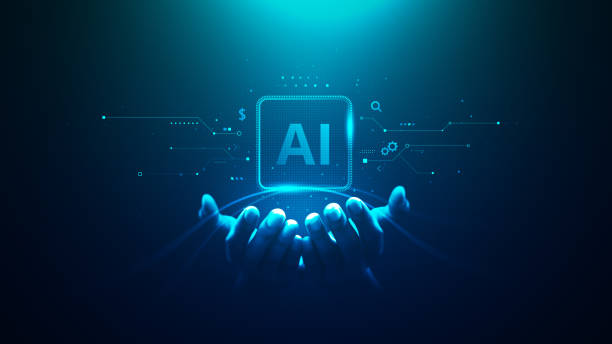
One of the most remarkable features of GPT-4 is image processing which helps in the visual analysis of data. Thanks to its sophisticated, deep neural network structure, the model is capable of recognizing, segmenting and enhancing images. This allows businesses and researchers to use GPT-4 for complex image analyses, ranging from marketing and advertising to medical diagnostics. For example, GPT-4 can assist in the evaluation of medical imaging studies, allowing for easier detection of abnormalities by the radiologists. Furthermore, its ability to create images can assist in brainstorming sessions for creative projects as designers can easily and quickly visualize their ideas.
Image Processing Features | Applications |
---|---|
Image Recognition | Healthcare diagnostics, Security systems |
Image Generation | Creative design, Advertising |
Image Enhancement | Forensics, Restoration of images |
The applications of GPT-4’s image processing abilities are diverse and transformative. Here are some prominent areas where these capabilities are making a significant impact:
- Healthcare: From diagnosing conditions using imaging techniques to enhancing the quality of medical images for better analysis.
- Marketing: Leveraging consumer behavior insights through image recognition to tailor advertisements effectively.
- Entertainment: In the creative fields, generating art or assisting in the visual effects process for films and games.
Harnessing Video Processing with GPT-4
Besides excelling in image processing, GPT-4 has impressive video processing skills. It is capable of deriving useful insights and creating content that meets user requirements by comprehending the context of video data. This means that video scenes can be understood as well as summarized, allowing the model to condense lengthier content to its essentials. The AI’s ability to automatically generate video content or captions is enhanced by sophisticated algorithms. This functionality can be used by video surveillance systems to easily identify anomalies or follow movements within a certain area, for example.
There is no shortage of possibilities when it comes to real-world use cases for GPT-4’s video processing capabilities. Here are just a few of them:
- Surveillance: Using AI to enhance security by monitoring and analyzing footage in real-time.
- Content Creation: Automating video editing processes, generating summaries, or creating highlights for sports and other events.
- Education: Developing interactive video content for enhanced learning experiences, such as adaptive learning environments.
Advantages of Multimodal Capabilities in GPT-4
Within the fundamentals of GPT 4 lies the capability of image and video processing. This feature alone brings in a host of benefits. To begin with, understanding context as a whole in terms of time and interaction improves. By working on different aspects at the same time, GPT 4 is able to produce responses that integrate various human senses which makes communication easier. This improves user experience while making responses to queries more accurate. Moreover, the amalgamation of these features caused the model’s ability to learn from different datasets to vastly improve leading to more diversity in understanding and less contextual biases.
Challenges in Multimodal AI
Although GPT-4 has many advancements, it still encounters some issues pertaining to multimodal AI. One problem is data accuracy. The model works best when it has access to high-quality images and videos during its training. This can oftentimes be challenging, particularly in more dynamic settings where the data is likely to change dramatically. Another problem involves bias in the training datasets, which can produce undesirable results. Additionally, the computational costs that come with handling multimedia content can be quite high, needing sophisticated equipment and software optimization. Overcoming these problems will be essential for the efficient use and integration of multimodal artificial intelligence systems.
Future Trends in Multimodal AI
The development of multimodal AI, especially with advances like GPT-4, is likely to be the most innovative areas of growth AI systems. Improvements in the power of neural networks and machine learning algorithms will also improve processing of images and videos. AI systems will be used more in augmented reality (AR) and virtual reality (VR) for offering more blended real and digital worlds experiences. Moreover, the growth of integrative AI systems which utilize the best of many models may bring extraordinary breakthroughs in different domains. Future healthcare, marketing, and entertainment will become more profound as researchers pursue these changes.
Conclusion
From this, we can infer that it is a leap forward in the improvement of multimodal artificial intelligence and the processing of images and videos, which can alter many fields. It can be utilized in many sectors such as providing health care services, diagnostics, and even automating video editing tools which shows how well it understands and produces multimedia content. The future of advancements is much more promising as the problems of data quality and biases are taken care of. The scope of multimodal AI is immense and it is foreseen that a lot of advancements will be made with great outcomes, one of which is the remarkable progression seen in the with artificial intelligence multimedia GPT-4.
Frequently Asked Questions
- What is multimodal AI? Multimodal AI refers to artificial intelligence systems that can process and analyze multiple forms of data simultaneously, such as text, images, and videos.
- How does GPT-4 process images? GPT-4 processes images using advanced algorithms that allow it to recognize, generate, and enhance images by understanding their context and content.
- Can GPT-4 generate videos? Yes, GPT-4 has the capability to analyze video content and generate relevant summaries or highlights, although the quality of generated video content can vary.
- What are the main applications of GPT-4’s image and video capabilities? Applications include healthcare diagnostics, marketing analysis, video surveillance, educational tools, and content creation across various platforms.
- What challenges does GPT-4 face in multimodal processing? Challenges include managing the large amounts of data required for training, addressing inherent biases, and ensuring data quality for effective analysis.